Dr.ssa Paola Antonini, Meditrial scientific director
The International medical device regulators forum (Imdrf), describes Software as a Medical Device (SaMD) as software that can run on generic (non-medical) computing platforms. They can be used in conjunction with other medical devices or other hardware/software. SaMD, which itself is a medical device, is one of three types of software related to medical devices. The other two types include software that is an integral part of a medical device and software used in the manufacture or maintenance of a medical device. SaMDs represent a revolution in digital health technology that can perform complex medical functions, diagnose conditions, indicate treatments and support clinical management. The use of software as a medical device continues to grow. SaMDs can be used for many purposes, including imaging and monitoring specific medical functions or parameters, aiding the health care provider in the diagnostic-therapeutic pathway.
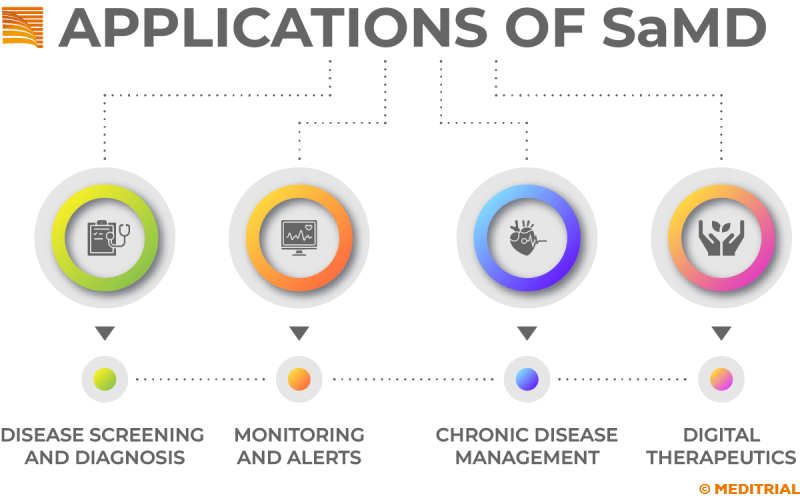
SaMD: REGULATORY PANORAMA
Given the unique characteristics of software as a medical device, which go beyond those of a traditional medical device or hardware, regulatory authorities have recognized the need to converge on a framework of common principles, enabling all stakeholders to promote innovation and protect patient safety. In 2013, the IMDRF established the Software as a Medical Device Working Group (WG) to develop guidelines to support innovation and timely global access to safe and effective SaMDs. Chaired by the FDA, the SaMD-related WG agreed:
- key definitions for SaMDs;
- The quality management system to be applied to SaMDs;
- The clinical evaluation of SaMDs;
The Imdrf Software as a medical device working group (WG) has therefore published a framework on the risk classification of SaMDs (SaMD: Possible framework for risk categorization and corresponding considerations). The recommendations provided in this document enable manufacturers and regulators to more clearly identify SaMD risk categories. These categories are based on the potential levels of impact the device output may have on the patient or public health. The document published by IMDRF classifies SaMDs into four different risk categories.
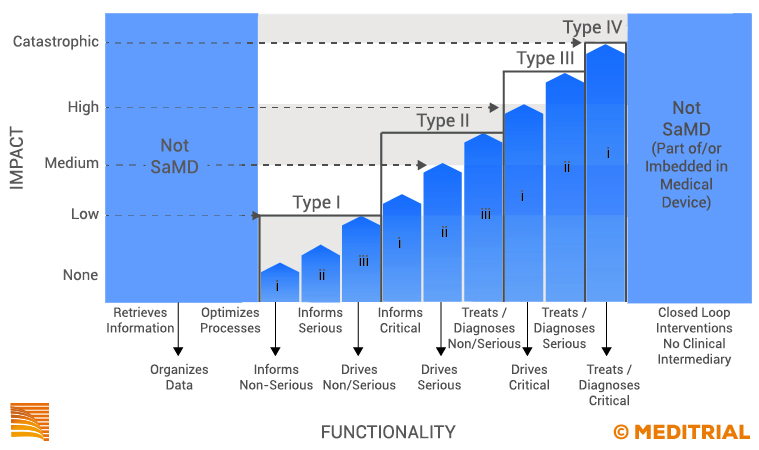
Device classification is, therefore, closely related to the criticality of the information provided by the SaMD to treat, diagnose, guide, or inform the clinical procedure. The accuracy of such data can be critical to avoid harmful health effects. The Level IV category is the SaMD with the greatest impact on the patient or public health and Level I is for the least impact.
A second document published by Imdrf is on the quality management of SaMDs (SaMD: Application of Quality management system). This document helps manufacturers and international regulatory authorities achieve greater understanding and a common language for the application of quality management system requirements to be applied to SaMDs throughout their life cycle. This document provides harmonized quality management principles that the Fda, along with other regulatory bodies, can adopt within their own regulatory frameworks.
Finally, it is worth noting that health care decisions increasingly use on the information provided by the SaMD. For these reasons, The Fda published the guideline: SaMD: Clinical evaluation – Guidance for Industry and Food and drug administration staff to describe the principles and approaches to be taken in the clinical investigation to demonstrate the safety, efficacy, and performance of software as a medical device. The guidance focuses on the activities to be undertaken by SaMD manufacturers for appropriate clinical evaluation of their software as a medical device.
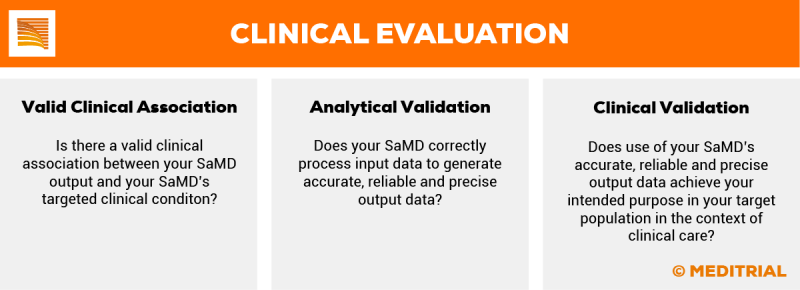
Artificial intelligence (Artificial Intelligence-AI) has been defined as the science of making intelligent machines. Artificial intelligence can use a variety of techniques, such as models based on statistical data analysis, evolved systems that rely primarily on “if-then” relationships, and machine learning (Machine learning-ML).
Machine learning is an artificial intelligence technique that is used to design algorithms intended to learn and act on received data. Software developers can use machine learning to create algorithms that are “Locked,” whose function does not change over time, or “Adaptive” so that their behavior changes based on new data.
Some examples of artificial intelligence and machine learning technologies include, for example, an imaging system that uses algorithms to provide diagnostic information.
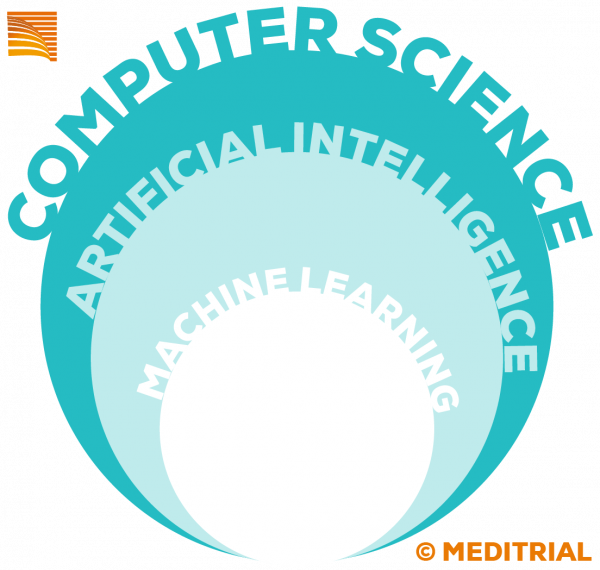
As a result, technology has rightfully entered every aspect of the health care system, enabling its rapid development. Software incorporating artificial intelligence, and in particular the subset of AI known as machine learning, has become a relevant part of increasing numbers of medical devices.
One of the major advantages of using AI/ML in software lies in its ability to learn from real-world use and experience and progressively improve performance. The new information obtained from SaMDs using AI/ML, therefore, can be a fundamental therapeutic diagnostic support.
Over the past decade, the Fda has reviewed and authorized an increasing number of devices incorporating AI/ML, with applications in various fields of medicine. Interest in medical devices incorporating ML functionality has thus increased, and this trend is expected to continue.
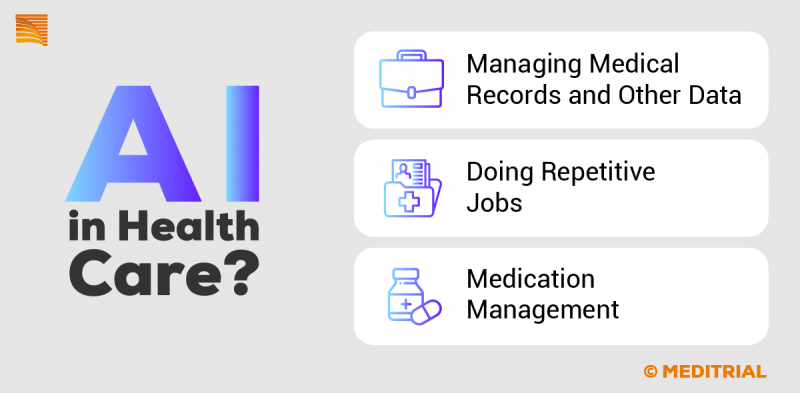
FDA GUIDING PRINCIPLES ON SaMDs
The use of AI and ML brings with it certain issues, such as the need to continually monitor, identify and manage the risks associated with these technologies, as well as implications pertaining to security, privacy and ethics.
Aiming to address these issues, in 2021, the Fda together with Health Canada and the U.K. Regulatory Agency (Mhra) jointly identified 10 guiding principles (Good machine learning practice for medical device development) to encourage and promote the development of safe, effective, and high-quality medical devices using AI and ML.
The Fda’s 10 guidelines cover a variety of issues, but many of them revolve around the technology development model, cybersecurity (cybersecurity) and risk mitigation requirements, as well as the need to involve as many experts as possible in the development and maintenance of SaMDs that rely on AI and ML.
These principles can help health systems that now rely on AI/ML medical devices to better understand and manage areas of criticality, as well as digital health startups and biotechnology companies to develop safe, effective, and high-quality AI/ML medical devices.
The guiding principles have as their primary goal the development of best practices for AI/ML technology development. However, The most challenging task for regulators and industry will be the development of targeted policies and procedures that fully integrate with existing quality regulations.
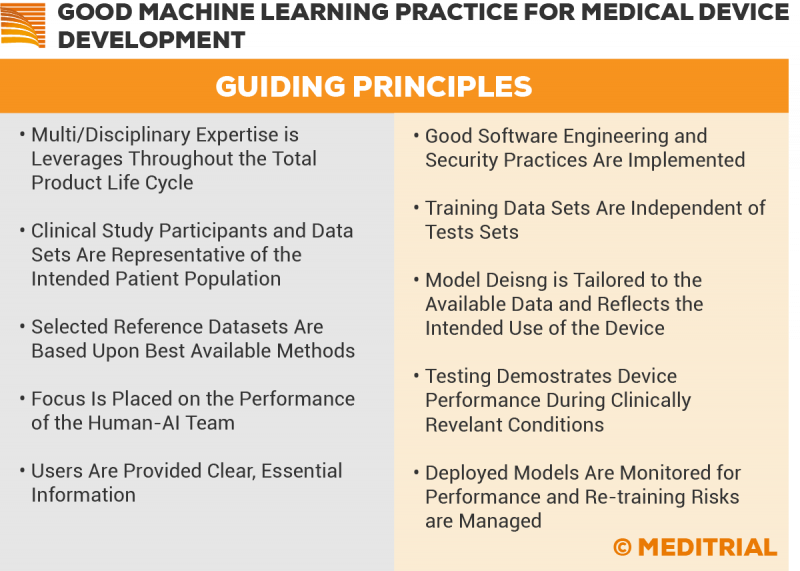
THE BASIC MODEL OF SaMDs
AI/ML-based medical devices have as their basic model a program or algorithm that is built and refined through the collection of a large amount of data. The model makes predictions based on the collected data. If the predictions are correct, the model learns and strengthens while if it is incorrect it adjusts to increase its accuracy. This learning process takes time and millions or billions of corrections. The more the model is refined, the better it can make correct diagnoses and reduce the number of incorrect ones.
The Fda’s 10 guiding principles help to ensure that the algorithms selected for the model are those best suited to the characteristics of the data collected and that the parameters are properly modified to produce the expected results.
The Fda recommends that the data collected for the model be representative of the expected patient population, that the model refer to clinically relevant data, and that the samples be large enough and of sufficient quality to allow experts to obtain detailed and accurate information. All this requires the active participation of various experts with multidisciplinary expertise to ensure that the model is sufficiently robust.
The Fda guidelines stress the importance of implementing robust cybersecurity practices, paying attention to the “fundamentals,” including basic software engineering practices.
Testing should verify device performance under clinically relevant conditions, and this requires a statistically sound test plan to ensure that data quality is intact and complete. Data authenticity and integrity must be ensured, not only during the design process but also after device implementation. In fact, continuous real-world device monitoring can improve security and performance and reduce bias and risk.
SaMD DEVELOPMENT IN THE HEALTH SECTOR
The implementation of AI/ML in healthcare is not easy to implement, requires significant investment and the collaboration of a large number of stakeholders and experts, which may require continuing education of both healthcare professionals and patients using these devices. However, the outcome in the use of these technologies can only lead to better monitoring of the patient’s clinical status.
Artificial intelligence and machine learning technologies have the potential to transform health care by drawing important new insights from the vast amount of data generated during everyday health care delivery. Medical device manufacturers are already using these technologies to innovate their products to assist healthcare providers and improve patient care.
Clinical performance studies should always be designed to specify the clinical evidence that the study is intended to produce taking into account potential risks, appropriate ethical requirements, and ensuring compliance with all applicable legal and regulatory requirements.
Through the Clinical Performance Study Plan (CPSP), the criteria, objectives, design, proposed analysis, methodology, monitoring conduct, and registration of the clinical performance study are defined (Annex XIII IVDR 2.3.2).
Clinical performance studies must always be designed to specify the clinical evidence the study is intended to generate taking into account potential risks, appropriate ethical requirements, and ensuring compliance with all applicable legal and regulatory requirements. Because clinical performance studies must be tailored to the specified intended population, the manufacturer must evaluate and justify the use of any sample within its performance study in consideration of the intended purpose and type of IVD. The substantial difference between the world of medical devices and in vitro diagnostic medical devices is the concept of “clinical performance” present only in the latter. The clinical performance of an in vitro diagnostic is its ability to produce results that are closely related to a particular clinical, psychological or pathological condition depending on its intended use (the ability of a test to detect the positivity/negativity of a patient).
Dr.ssa Paola Antonini, Scientific Director Meditrial
Leave A Comment